Retrieval-Augmented Generation (RAG) has revolutionised the way we approach GenAI. By merging the capabilities of information retrieval and GenAI, RAG has set new benchmarks in performance and application. This blog post will explore the key milestones in the development of RAG and its evolution compared to traditional NLP methods.
The Genesis of RAG š
The concept of RAG began to take shape around 2020, primarily through the work of Lewis et al. in their groundbreaking paper titled "Retrieval-Augmented Generation for Knowledge-Intensive NLP Tasks." This paper introduced a novel architecture that utilized both parametric and non-parametric memory for language generation, allowing models to retrieve relevant information from external databases like Wikipedia before generating text. This combination significantly improved the accuracy and relevance of generated outputs, setting RAG apart from traditional NLP models, which often relied solely on learned parameters and could suffer from inaccuracies or "hallucinations" (when models generate incorrect or fabricated information).
The Role of Pre-trained Models š§
The rise of RAG was also facilitated by the earlier successes of large pre-trained models, particularly BERT and GPT. These models laid the groundwork for RAG by showcasing the potential of combining extensive knowledge from pre-training with generative capabilities. RAG further capitalizes on this synergy, effectively bridging the gap between retrieval and generation.
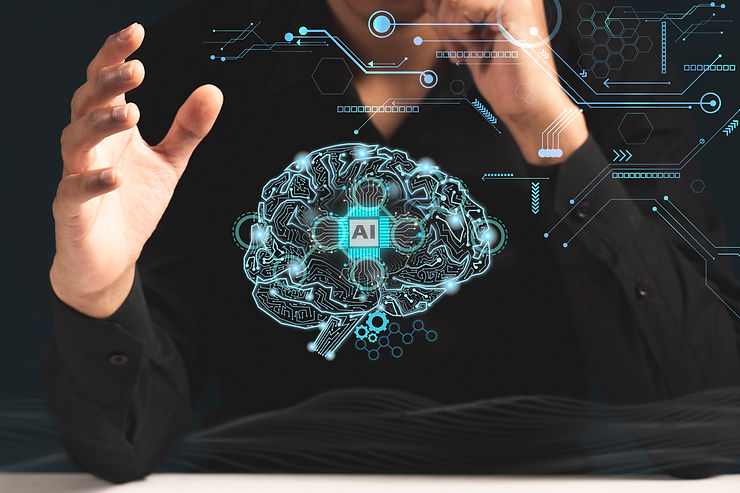
Architectural Innovations šļø
RAG architecture comprises several unique features that differentiate it from traditional NLP methods. For instance, the integration of dense retrieval techniques allows RAG to utilize vector embeddings to find semantically relevant documents efficiently. Compared to traditional sequence-to-sequence models, which often struggle with contextually rich queries. Moreover, RAG's multi-step reasoning capabilities enhance its ability to refine outputs iteratively, making it particularly useful for complex queries that require specialized knowledge.
Key Milestones in RAG Development š
2020 ā Initial RAG Models: The introduction of RAG by Lewis et al. marked a significant leap in NLP. Their experiments demonstrated that RAG could outperform existing state-of-the-art models in various knowledge-intensive tasks.
2021 ā Further Innovations: New variants of RAG, such as HyDe (Hypothetical Document Embedding) and Self-RAG, emerged. These models improved upon the original framework by allowing for hypothetical document generation and iterative query refinement.
2023 ā Widespread Adoption: As RAG models evolved, they began to see practical applications across industries, from automated customer service to content generation, showcasing their versatility and efficiency.
The Future Landscape of RAG š
Looking ahead, RAG is poised to address critical challenges, particularly in ethical considerations. Ensuring transparency and fairness in the training data will be paramount for its widespread adoption. Moreover, as advancements continue, we can expect RAG to further integrate with emerging technologies, enhancing its capability to provide contextually relevant and accurate information.
For more in-depth studies on RAG, check out the following:
Lewis, M., et al. "Retrieval-Augmented Generation for Knowledge-Intensive NLP Tasks." arXiv:2005.11401
Letās embrace the future of RAG together! šāØ
Comments